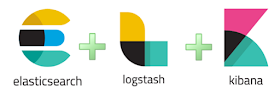
how to see the application logs and try to find problems. Because the application logs can be distributed on many machines, as we can see in the figure below:
So from the outset of the project the logs should be a concern, as the project grows the trail of errors and success should be easily found. Because depending on the organization a mistake can cost a lot of money or even stop a business operation for a few hours causing a lot of damage.
A good stack that I have been using in the projects I have been working on is the ELK stack or Elastic Stack that is based on 3 main components, but I consider 4 because there is one in this very useful scenario:
- Elastic Search
- Logstash
- Kibana
- FileBeat
Elasticsearch is a highly scalable full-text search and analysis engine. It allows storing, searching and analyzing large volumes of data quickly and in near real time.
RESTful distributed search and analysis tool capable of solving a growing number of use cases. Like the heart of the elastic pile, it centrally stores your data so you can discover what's expected and discover the unexpected.
Logstash is a processing pipeline that ingests data from a multitude of sources at once, transforms it, and then sends it to ElasticSearch (in that case because it can send to other databases as well).
Data is usually scattered or spread across many systems in various formats. Logstash supports a variety of entries that draw events from multiple common sources all at the same time. Easily ingest your logs, metrics, web applications, data storage and various services.
As the data arrives, the Logstash filters analyze each event, identify the named fields to build the structure, and transform them to converge in a common format for analysis.
Kibana allows you to view your Elasticsearch data and navigate the data, creating filters, aggregations, count, combinations, that is, a visual way of navigating the data that is stored in ElasticSearch.
With Kibana you can create graphs of various types eg:
Filebeat helps keep things simple by offering a lightweight way to forward and center logs and files. Instead of making a tail in the file machine, the fileBeat agent does it for us.
In each machine where there is service is installed a fileBeat agent that will be in charge of observing the logs and forwards to its configured Logstash.
Instalação:
To configure this stack in the initial mode we can choose to have only one machine where we will put ElasticSearch, Logstash and Kibana.
NOTE: In the example below I am using a CentOS operating system.
ElasticSearch installation:
#sudo rpm --import https://artifacts.elastic.co/GPG-KEY-elasticsearch
create folder /etc/yum.repos.d/ and create file elasticsearch.repo
and add in file elasticsearch.repo these content:
[elasticsearch-5.x]name=Elasticsearch repository for 5.x packagesbaseurl=https://artifacts.elastic.co/packages/5.x/yumgpgcheck=1gpgkey=https://artifacts.elastic.co/GPG-KEY-elasticsearchenabled=1autorefresh=1type=rpm-md
#sudo yum install elasticsearch
if everthing ok this command curl -XGET 'localhost:9200/?pretty' should return on Json with default content.
Logstash installation:
Fist Should be installed JAVA 8 or JAVA 9.
#sudo rpm --import https://artifacts.elastic.co/GPG-KEY-elasticsearch
create folder /etc/yum.repos.d/ and create file logstash.repo
and add in file logstash.repo these content:
[logstash-5.x]name=Elastic repository for 5.x packagesbaseurl=https://artifacts.elastic.co/packages/5.x/yumgpgcheck=1gpgkey=https://artifacts.elastic.co/GPG-KEY-elasticsearchenabled=1autorefresh=1type=rpm-md
#sudo yum install logstash
With these tools working, we can already configure the stack to start ingesting data in ElasticSearch.
The first tool to be configured is Logtash.
Configuration:
Logstash:
In the logstash config folder the INPUT, FILTER, and OUTPUT must be configured for the files that will be consumed in my example:
input {beats {port => "5043"}}filter {grok {match => { "message" => "\A%{TIMESTAMP_ISO8601}%{SPACE}%{LOGLEVEL}%{SPACE}%{INT}%{SPACE}%{SYSLOGPROG}%{SPACE}%{SYSLOG5424SD}%{SPACE}%{JAVACLASS:service}%{SPACE}%{NOTSPACE}%{SPACE}%{JAVALOGMESSAGE:java_message}"}}grok {match => { "message" => "\A%{TIMESTAMP_ISO8601}%{SPACE}%{SYSLOG5424SD}%{CRON_ACTION}%{JAVACLASS:servico}%{CRON_ACTION}%{SYSLOG5424SD}%{SPACE}%{JAVALOGMESSAGE:java_message}"}}}output {elasticsearch {hosts => [ "localhost:9200" ]index => [“my-example"]}}
After this configuration is applied, Logstash must be restarted.
FileBeat:
The agent must be configured machine by machine, a task that can be made easier using the ANSIBLE, which is triggered from only one machine adding the agent in the others:
Example, I created the file playbook-filebeat.yml, inside it has installation and configuration commands.
- hosts: "{{ lookup('env’,'HOST') }}"vars:http_port: 80max_clients: 200remote_user: my-userenvironment:AWS_ACCESS_KEY_ID: MYKEYAWS_SECRET_ACCESS_KEY: MYSECRETtasks:- name: Stop FileBeat if runningbecome: yesbecome_method: sudoshell: '/etc/init.d/filebeat stop'ignore_errors: yes- name: FileBeat downloadshell: "curl -L -O https://artifacts.elastic.co/downloads/beats/filebeat/filebeat-5.2.2-x86_64.rpm"ignore_errors: yes- name: FileBeat Installbecome: yesbecome_method: sudoshell: "sudo rpm -vi filebeat-5.2.2-x86_64.rpm"ignore_errors: yes- name: Install Pip with Curl and Pythonget_url: url=https://bootstrap.pypa.io/get-pip.py dest='/home/ec2-user/'ignore_errors: yes- name: execute install scriptbecome: yesbecome_method: sudoshell: 'python /home/ec2-user/get-pip.py'ignore_errors: True- name: install aws clibecome: yesbecome_method: sudoshell: 'pip install --upgrade --user awscli'- name: get script from s3become: yesbecome_method: sudoshell: '~/.local/bin/aws s3 cp s3://scripts/filebeat.conf-{{ENV}}.yml /etc/filebeat/filebeat.yml --region sa-east-1'- name: Stop FileBeat if runningbecome: yesbecome_method: sudoshell: '/etc/init.d/filebeat start'ignore_errors: yes
You can run this playbook with this command:
#ansible-playbook playbook-conf/playbook-filebeat.yml --private-key my_pem_file.pem -e "HOST"=my.service.host
Details on the use of ANSIBLE in this post: http://salerno-rafael.blogspot.com.br/2017/01/automating-infrastructure-on-premise-or.html
To avoid entering the machine and adding the configuration of FileBeat I put my configuration file in S3 and from inside the machines I look for this file.
FileBeat configuration file to be added in /etc/filebeat/filebeat.yml
filebeat.prospectors:- input_type: logpaths:- /logs/service1/service.logoutput.logstash:hosts: ["logstash-host:5043”]
NOTE: If you do not want to use ANSIBLE, you can perform tasks manually.
With this structure running we can start consuming the application logs.
Example of Logs to be filtered by GROK pattern:
2017-07-04 11:11:37.921 INFO 60820 --- [pool-3-thread-1] br.com.service.ContaService : GET DATA2017-07-04 11:11:37.952 INFO 60820 --- [pool-3-thread-1] br.com.ContaServiceLog : CALL SOMEthing2017-07-04 11:11:37.954 INFO 60820 --- [pool-3-thread-1] br.com.ContaServiceLog : http://some-service
Now we have this structure working the structure according to the figure below:
The ELK stack is very powerful, from here we can create countless metrics, search, filters etc with the data that is inserted in ElasticSearch.
References :
- https://www.elastic.co
- https://www.digitalocean.com/community/tutorials/how-to-install-and-configure-elasticsearch-on-ubuntu-14-04
- http://www.javacodegeeks.com/2013/02/your-logs-are-your-data-logstash-elasticsearch.html
- http://logstash.net/docs/1.1.9/tutorials/10-minute-walkthrough/
- https://www.elastic.co/guide/en/logstash/current/configuration.html